
The language model can definitively decide that, in this context, the word “block” makes much more sense. This is because the acoustic model can often confuse two or more similar-sounding words.įor example, if it identifies that the speaker has just said: “I walked around the _” and it identifies that the next word should be either “clock” or “block” but it can’t decide which, then the acoustic model can defer to the language model for the final decision. While an ASR system could technically operate successfully without access to a language model, doing so highly limits its accuracy. RNNs are the tool of choice in language modeling, specifically things like LSTMs, GRUs, and Transformers. Normally language modeling is done at the word level, but it can also be done at the character level which is useful in certain situations such as for languages that are more character-based (Chinese, Japanese, etc.).
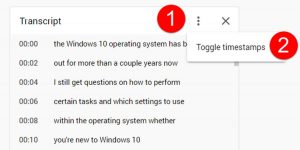
The core goal in language modeling is, given a sequence of words, to predict the next word in the sequence.

The second part of the automatic speech recognition system, the language model, originates in the field of natural language processing. Transfer learning is a relatively new idea that’s been used extensively in computer vision and NLP and has now made its way to the speech recognition field. This process involves taking the pre-trained weights from the original model and fine-tuning them on a new dataset in the target language. However, if the two languages have some vocal similarities, as these two do, then engineers can use a technique called transfer learning to take the original model and transfer it to the new language. An acoustic model used for English, for example, can’t be used for German. It has access to training data from years of work of 50,000 native English-speaking transcriptionists and the corresponding audio files.Īcoustic models also differ between languages. This is one reason why Rev’s automatic speech recognition excels. This is why it’s so important for the acoustic model to have access to a huge repository of (audio file, transcript) training pairs. Thus, while a naively trained acoustic model might be able to detect well-enunciated words in a crystal clear audio track, it might very well fail in a more challenging environment. The exact sounds used and the exact characteristics of the waveform depend on a number of variables such as the speaker’s age, gender, accent, emotional tone, background noise, and more. Part of what makes acoustic modeling so difficult is that there is huge variation in the way any single word can be spoken. These include the Hidden Markov Model, Maximum Entropy Model, Conditional Random Fields, and Neural Networks. Many different model types have been used over the years for the acoustic modeling step. They provide a way of identifying the different sounds associated with human speech. Phonemes are like the atomic units of pronunciation.

The waveform is typically broken into frames of around 25 ms and then the model gives a probabilistic prediction of which phoneme is being uttered in each frame. This model takes as input the raw audio waveforms of human speech and provides predictions at each timestep. The Acoustic ModelĪs mentioned, one of the key components of any ASR system is the acoustic model. This, along with the raw acoustic waveforms and the words they suggest, allow models to accurately transcribe speech. The RNN architecture allows the model to attend to each word in the sequence and make predictions on what might be said next based on what came before. RNNs are useful because they were designed to deal with sequences, and human speech involves sequences of word utterances. Try the Rev AI Speech Recognition API FreeĬurrent speech-to-text models rely heavily on recurrent neural networks along with a few other tricks thrown in.
